Whether the person is your biggest fan or a first-time customer you know nothing about, recommendations may be implemented at each stage of the customer journey. One kind of suggestion could persuade a browser to make a purchase, whilst another kind might assist foster loyalty after a sale.
In either case, product recommendation algorithm are your friend. In our most recent guide, we’ve outlined six variations you should be familiar with, where to apply them, and which businesses are leading the way.
What is a product recommendation?
Product recommendations are a component of an eCommerce customization strategy in which goods are dynamically provided to a user on a webpage, app, or email based on data such as customer traits, browsing activity, or situational context, resulting in a personalized purchasing experience.
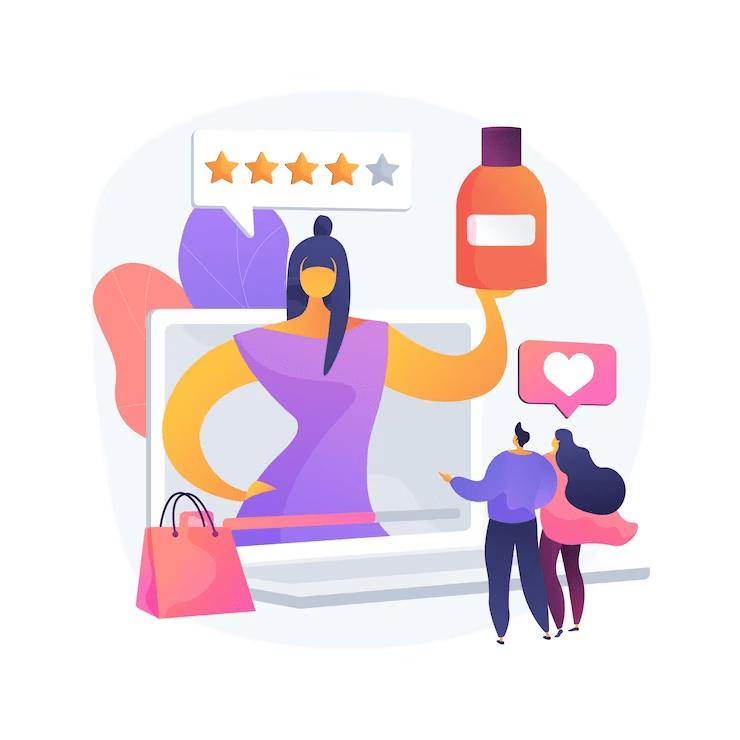
Product suggestions are especially useful for firms with a broad and diversified product portfolio. The catalog may be diversified owing to a vast range of items (e.g., a department store), or it may contain a small number of product categories but a wide range of product feature sets (e.g. an eyeglass retailer).
Best product recommendation algorithm for BigCommerce stores
Trending topics
The trending algorithm, like popular suggestions, focuses on a brand’s greatest sellers, but only within a specific time window. If you launch a new product that quickly becomes popular, the trending recommendation system will prefer it over the constant top sellers.
Popular
Popular product recommendation algorithms promote goods that are, you guessed it, the most popular. These are the best-selling items throughout a brand’s whole inventory, and they do not take into account individual consumers’ unique interests and data, nor where they may be along the customer journey.
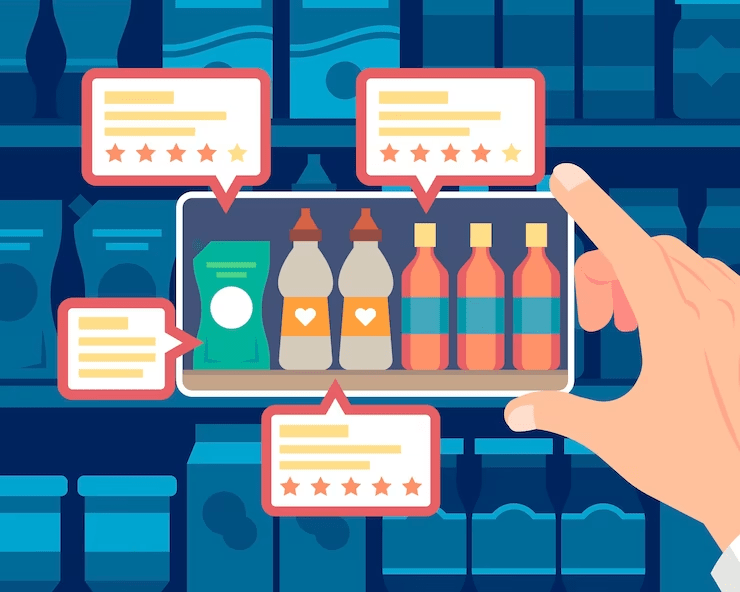
Contextual
Contextual recommendations fall under the umbrella of collaborative filtering, sometimes known as “Those who purchased this also bought that.” These recommendation algorithms require a single data set to function: the URL a consumer is visiting. They then use the wider site history to offer recommendations.
Browsing history
Collaborative filtering is also used in recommendations based on browsing history to identify products that have prompted consumers with similar records to make purchases. These recommendations, which are great for product discovery, may be applied to users who have only generated two-page visits. Nonetheless, extended browsing histories may enhance their usefulness.
Purchase history
These product recommendation algorithms demand more information from marketers about their clients, especially what they have previously purchased. Purchase history uses a much stronger signal since individuals explore a lot of things they don’t buy, which eventually makes it a high-quality suggestion.
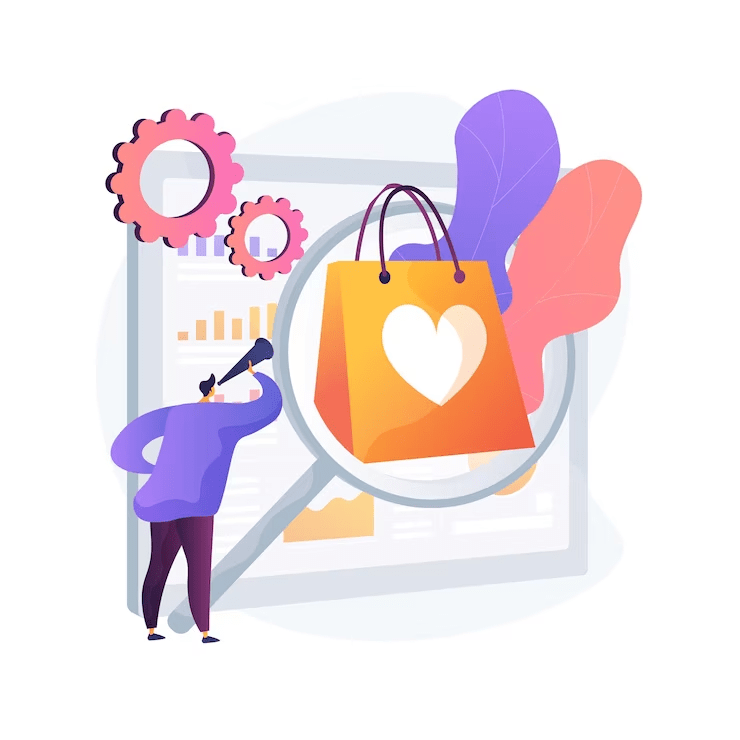
Interest-based
Every page a client visits is recorded by interest suggestion algorithms as they browse, adding interest tags for that material to each user’s profile. The algorithms use a customer’s interest tags, which are updated in real-time as they interact with the brand, to match products with those tags.
In conclusion,
Do you wish to provide product recommendations based on a use case not covered above? Any of these may be combined with custom suggestions and any other product recommendation algorithms your business may have already developed. The globe is open to you. Our product recommendation app for BigCommerce – OrderBooster can help you get started the right way!