In today’s rapidly evolving world of eCommerce, personalized shopping experiences have become the cornerstone of success for businesses. With the advent of machine learning product recommendation systems, companies can now harness the power of advanced technology to deliver tailored and engaging experiences to their customers. Machine learning product recommendation aims to make the shopping experience seamless and effortless for customers. By presenting them with products that align with their needs and preferences, businesses can reduce the time and effort required for customers to find what they want.
Advantages of Machine Learning-Based Recommendations Over Traditional Methods
Machine learning-based recommendations offer several advantages over traditional methods, revolutionizing how businesses personalize and optimize the shopping experience for their customers. Here are some key benefits:
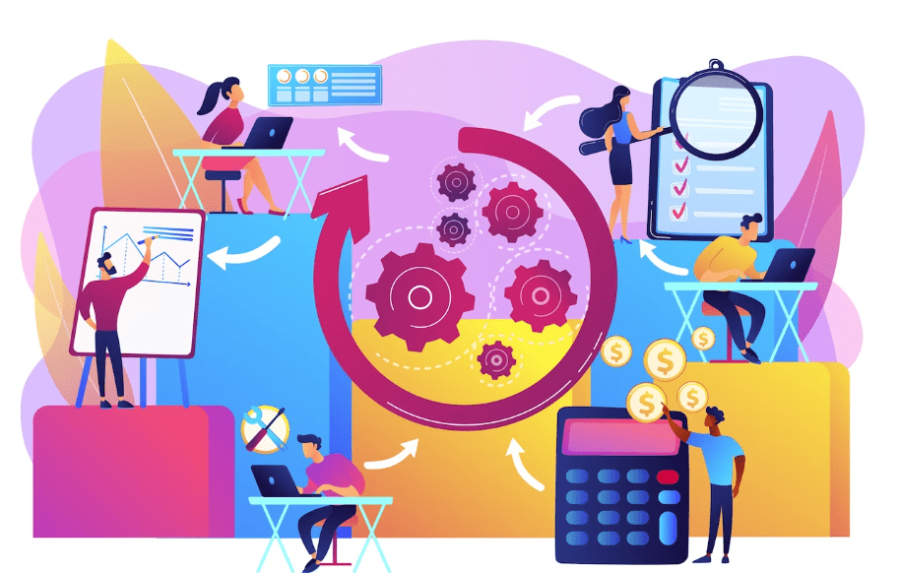
Enhanced Accuracy: Machine learning algorithms can analyze vast customer data, including browsing history, purchase patterns, and demographic information, to generate highly accurate and relevant recommendations. This level of precision surpasses traditional methods, which often rely on manual rules or simplistic algorithms.
Personalization at Scale: Machine learning allows businesses to personalize recommendations at scale. As the volume of data increases, the algorithms adapt and improve, ensuring that each customer receives tailored suggestions based on their unique preferences. Traditional methods need help to provide such individualized experiences on a large scale.
Real-Time Adaptation: Machine learning algorithms continuously learn and adapt based on real-time customer interactions. They can quickly respond to changes in customer preferences and market trends, ensuring that the recommendations remain up-to-date and relevant. Traditional methods typically need more agility and responsiveness.
Discoverability of New Products: Machine learning algorithms can identify patterns and correlations in customer behavior, enabling them to suggest products that customers may not have discovered independently. This helps businesses promote new or less-known items, increasing exposure and driving sales.
Key Components of Machine Learning Product Recommendation Systems
Data collection and preprocessing
The foundation of any machine learning system is data. In the context of product recommendation, customer data such as browsing history, purchase history, and demographic information is collected and preprocessed. This includes cleaning the data, handling missing values, and transforming it into a format suitable for analysis.
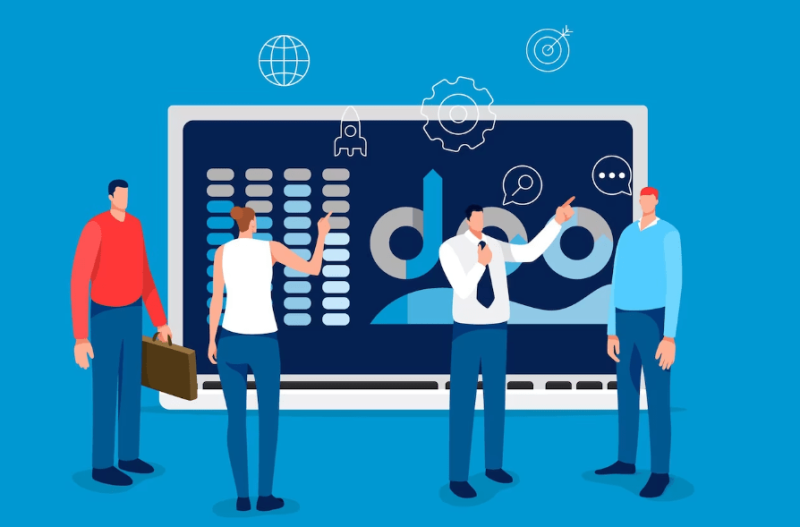
Algorithm selection and model training
Machine learning algorithms are crucial in generating accurate and relevant recommendations. Various algorithms can be employed, such as collaborative filtering, content-based filtering, and hybrid approaches. These algorithms are trained using historical customer data to recognize patterns, similarities, and preferences. The models are continuously refined and optimized to improve the accuracy of the recommendations.
Real-time recommendation generation
Machine learning product recommendation systems operate in real-time, analyzing user interactions and generating personalized suggestions on-the-fly. As customers navigate an eCommerce website, the system dynamically adapts and updates the recommendations based on their current behavior. This real-time aspect ensures that the recommendations are tailored to the individual customer’s needs and preferences at that specific moment.
Conclusion
Machine learning-based product recommendation systems are transforming the landscape of eCommerce, offering numerous advantages over traditional methods. Don’t hesitate to get in touch with us to learn more about how BigCommerce can help you implement cutting-edge machine learning product recommendation systems and optimize your eCommerce strategy for success.